The Best Fluffy Pancakes recipe you will fall in love with. Full of tips and tricks to help you make the best pancakes.
Table of Contents
Introduction to AI in Finance

Artificial Intelligence (AI) has emerged as a transformative force in numerous sectors, with the financial industry being at the forefront of this change. The integration of AI technologies in finance dates back to the 1980s, when basic algorithms were developed to facilitate data analysis. However, it is within the last two decades that AI has made significant strides, harnessing advancements in machine learning, natural language processing, and big data analytics. This evolution has enabled financial institutions to process vast amounts of information and derive insights that were previously unattainable.
The early applications of AI in finance were mainly focused on improving efficiency in routine tasks, such as data entry and transaction processing. Over time, as computational power increased and data became more accessible, the capabilities of AI have expanded dramatically. learn: how to Make Money with AI Artificial Intelligence in 2025; Today, AI is not only enhancing operational efficiencies but also playing a pivotal role in risk assessment, fraud detection, trading algorithms, and personalized financial services. For instance, machine learning models can analyze market trends and consumer behavior to generate actionable investment strategies, which are increasingly favored over traditional methods.
Moreover, AI’s ability to learn from vast datasets allows financial institutions to quickly adapt to changing market conditions. Predictive analytics tools can forecast market movements with a higher degree of accuracy, enabling investors to make informed decisions. Furthermore, chatbots and virtual assistants powered by AI are revolutionizing customer service, offering tailored financial advice and support in a user-friendly manner. This technological advancement signals a paradigm shift, where data-driven insights are replacing intuition-based decision-making.
The continuous development of AI technologies in finance indicates a strong trajectory toward increased automation and personalization. As we delve deeper into the impact of AI on investment strategies, it is clear that the financial landscape is undergoing a significant transformation, laying the groundwork for more sophisticated, efficient, and customer-centric practices.
The Role of Machine Learning in Investment Strategies
Machine learning, a prominent subset of artificial intelligence, has transformed the landscape of investment strategies in recent years. By leveraging advanced algorithms, machine learning systems can analyze vast amounts of financial data, identifying hidden patterns and trends that may not be immediately apparent to human investors. This capability enhances decision-making processes, enabling more informed and data-driven investment choices.
In the realm of finance, machine learning models employ various techniques, including supervised and unsupervised learning, to process historical market data. By training on time-series data, these algorithms detect correlations and fluctuations in asset prices, which serve as valuable indicators for predicting future market movements. For instance, machine learning can assess economic indicators, corporate earnings, and even social sentiment to forecast stock performance.
Moreover, the ability of machine learning to continuously learn from new data allows investment strategies to adapt to evolving market conditions. Algorithms can refine their predictions over time, incorporating fresh information, thereby enhancing their accuracy and efficacy. This adaptability is particularly crucial in today’s rapidly changing financial environment, where shifts in market trends can occur swiftly.
Investment firms increasingly utilize machine learning to optimize trading strategies and portfolio management. By employing predictive analytics, these firms can automate trading decisions, manage risk more effectively, and identify lucrative investment opportunities with higher precision. Additionally, machine learning facilitates the diversification of portfolios by evaluating a multitude of options and weighing potential risks versus rewards.
Overall, the integration of machine learning into investment strategies marks a significant evolution, providing investors with advanced tools that help enhance performance and reduce uncertainties. As the technology continues to progress, its impact on the finance industry will likely grow, further shaping the future of investing.
Algorithmic Trading: Speed and Efficiency

Algorithmic trading refers to the use of computer algorithms to execute trades in financial markets with high speed and efficiency. Leveraging Artificial Intelligence (AI) technologies, this method allows traders to analyze market data and trading patterns much faster than traditional methods. The automation of trading strategies means that decisions can be executed in fractions of a second, capitalizing on fleeting opportunities in the markets that a human trader might miss.
One of the primary benefits of algorithmic trading is its ability to process vast amounts of data in real time. AI algorithms can analyze historical price movements, market indicators, and macroeconomic factors to generate trading signals. By using sophisticated statistical models and machine learning techniques, these algorithms continually improve their strategies, adapting to changing market conditions. As such, they can identify highly profitable trades and optimize execution timing, greatly enhancing market efficiency.
Moreover, algorithmic trading reduces the emotional aspect often associated with trading decisions. By relying on predefined criteria, these systems minimize human error and emotional responses to market volatility, leading to more disciplined and objective trades. This automation not only increases precision but also improves the overall liquidity of financial markets, making it easier for participants to enter and exit positions swiftly.
However, there are potential risks associated with algorithmic trading. Flash crashes, caused by rapid automated trading, can lead to significant market disruptions. When multiple algorithms respond to similar market signals simultaneously, it can result in extreme price swings within a very short time frame. Additionally, there are concerns regarding market manipulation and the creation of unfair advantages for certain traders utilizing sophisticated AI technologies. Thus, while algorithmic trading presents remarkable opportunities for enhanced efficiency, careful consideration of its risks is essential to ensure market stability.
Predictive Analytics and Market Forecasting
Predictive analytics has emerged as a crucial component in financial forecasting, radically transforming how investors approach market analysis. Utilizing sophisticated artificial intelligence models, predictive analytics leverages historical financial data to discern patterns and trends that can offer insightful predictions about future market movements. This analytical approach empowers investors to make data-driven decisions, enhancing their overall investment strategies.
AI-driven predictive analytics employs various statistical techniques and machine learning algorithms to analyze vast quantities of historical data. These methodologies include time series analysis, regression modeling, and neural networks, which enable the identification of potential market anomalies and shifts in trends. By processing real-time data coupled with historical context, predictive models can offer forecasts that are more accurate than traditional analysis methods.
Moreover, the application of predictive analytics is particularly advantageous for risk management. Investors can utilize its insights to identify potential downturns and adjust their portfolios proactively. This level of foresight allows for better capital allocation, significantly minimizing the potential for losses during volatile market conditions. Additionally, investors can better understand the factors driving market movements, whether they are macroeconomic indicators, sector trends, or geopolitical events.
As financial markets become increasingly complex and interdependent, the demand for tools that support informed decision-making rises sharply. Predictive analytics not only aids in understanding these complexities but also equips investors with the tools needed to seize profitable opportunities swiftly. By incorporating predictive analytic techniques into their investment processes, investors can enhance their ability to forecast market fluctuations and optimize their investment allocations accordingly.
Risk Management and Fraud Detection

Artificial intelligence is significantly transforming the financial landscape, particularly in the realms of risk management and fraud detection. By employing advanced algorithms and machine learning techniques, AI tools can analyze vast amounts of data at unprecedented speeds. This capability allows financial institutions to assess risk levels effectively, enabling them to make data-driven decisions that enhance overall investment security.
In terms of risk management, AI systems utilize predictive analytics to anticipate potential market fluctuations and identify underlying risk factors. They examine historical data, current market conditions, and various economic indicators to generate sophisticated risk profiles for different investments. This comprehensive assessment allows portfolio managers to align their strategies with their risk tolerance, ensuring that they can respond promptly to market changes. Such timely interventions can mitigate losses and enhance the overall stability of investment portfolios.
Fraud detection is another critical area where AI’s capabilities come into play. Financial institutions are increasingly deploying AI-driven solutions to monitor transactions in real-time. By leveraging machine learning algorithms, these systems can identify unusual patterns or behaviors that may indicate fraudulent activity. For instance, if a transaction deviates significantly from a user’s typical spending pattern, the AI system can flag it for further review, significantly reducing the time and resources spent on manual fraud detection processes.
Moreover, AI enhances the accuracy of fraud detection systems by continuously learning from new datasets. Each detection instance contributes to refining the algorithms, allowing them to adapt to emerging fraud tactics. As a result, AI tools not only increase operational efficiency but also help in establishing a more secure investment environment by significantly minimizing financial losses attributed to fraud.
Personalized Investment Experiences through AI
The integration of artificial intelligence (AI) in finance is revolutionizing the way investment experiences are tailored to individual clients. Financial institutions are increasingly leveraging AI technologies to analyze vast amounts of data and extract insights that enable personalized investment strategies. By utilizing machine learning algorithms and advanced analytics, these institutions can deliver investment solutions that align closely with each client’s unique preferences, goals, and risk appetites.
One significant way AI enhances personalization in investing is through the analysis of client behavior and financial history. By examining transaction patterns, investment choices, and even social media activity, AI can craft bespoke portfolios that reflect an investor’s past decisions and future aspirations. This tailors the investment experience, ensuring that clients receive recommendations that are not only relevant but also conducive to their financial objectives.
Moreover, financial advisors are utilizing AI-powered tools to engage with clients in a more meaningful manner. Chatbots and virtual assistants are capable of providing real-time advice based on the latest market trends and individual financial situations. As these AI systems evolve, they become adept at predicting market fluctuations and identifying opportunities that clients may not have considered, thus enhancing their overall investment proficiency. Furthermore, by offering a streamlined experience, financial institutions can reduce the friction often associated with making investment decisions.
Additionally, AI enables continuous improvement in personalized investment strategies. As clients’ financial situations and market conditions change, AI algorithms can quickly reassess and adjust recommendations, ensuring that investment strategies remain aligned with the evolving landscape. This dynamic approach ultimately leads to better investment outcomes, fostering client satisfaction and loyalty in the competitive finance sector.
The Impact of AI on Financial Advisors

The role of financial advisors is undergoing a significant transformation in the era of artificial intelligence (AI). Traditionally, financial advisors have been the primary source of investment advice and portfolio management for their clients. However, the advent of AI tools is reshaping this dynamic, enabling advisors to enhance their service offerings while simultaneously re-evaluating their relationships with clients. AI technologies can analyze vast amounts of data at unprecedented speeds, providing insights that allow financial advisors to make more informed decisions on behalf of their clients.
One of the key benefits AI brings to financial advising is the augmentation of human capabilities. AI tools, such as advanced analytics and predictive modeling, can assist advisors in understanding market trends and client behaviors better than ever before. This empowerment allows advisors not only to present data-driven recommendations but also to personalize investment strategies that align with an individual client’s objectives and risk tolerance. By leveraging AI, advisors can provide greater value and enhance the overall client experience.
Moreover, the integration of AI in the financial advising process can lead to a shift in client relationships. With AI handling many of the routine analyses and data management tasks, financial advisors can dedicate more time to building deeper relationships with clients. Instead of spending hours on number crunching, they can focus on understanding clients’ goals, fostering trust, and delivering tailored financial advice. This transition emphasizes the human element of financial advising, where emotional intelligence and personal connections become invaluable.
Furthermore, the competition between robo-advisors and traditional financial advisors is on the rise. Robo-advisors leverage algorithms to automate investment management at a lower cost, attracting a demographic that might previously have relied on human advisors. This competition forces traditional advisors to adapt and incorporate AI tools in their practice to stay relevant and competitive. Ultimately, the integration of AI into financial advising presents both challenges and opportunities, compelling financial advisors to evolve in their approach to client service and investment strategy.
Challenges and Ethical Considerations of AI in Finance
The implementation of Artificial Intelligence (AI) in finance has brought about a multitude of advancements, yet it is not without its challenges and ethical implications. One of the foremost concerns is data privacy. Financial institutions often handle vast amounts of sensitive personal information. As AI systems process this data to make investment decisions, there is a significant risk of data breaches or unauthorized access, which could jeopardize client confidentiality and trust.
Moreover, algorithmic bias presents another critical challenge in the domain of AI-driven finance. Algorithms require extensive datasets for training, and if these datasets are flawed or unrepresentative, the AI can produce biased outcomes. This bias may not only affect investment recommendations but could also perpetuate existing inequalities within the financial system, disadvantaging certain groups of individuals based on race, gender, or socio-economic status. Recognizing and mitigating these biases is essential to ensure fair and equitable financial practices.
Another aspect to consider is the accountability of AI-driven decisions. With algorithms making critical financial decisions, it can be unclear who bears responsibility when issues arise. For example, if an AI system makes a poor investment recommendation resulting in significant losses, identifying the accountable party—whether the developers, the financial institution, or the AI itself—can be complex. This ambiguity raises questions about the need for regulations to govern AI operations in the finance industry and to clarify liability in the event of mistakes.
Addressing these challenges is vital for the responsible advancement of AI in finance. Establishing robust regulatory frameworks can help protect consumer rights, maintain data privacy, and promote fairness in AI applications. As technology continues to evolve, ongoing discussions surrounding these ethical considerations will be essential to foster a balanced approach to AI in the financial sector.
The Future of AI in Investment Strategies
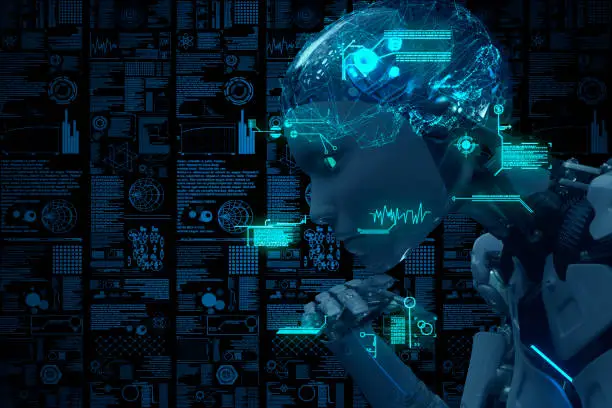
The integration of artificial intelligence (AI) in finance has significantly transformed investment strategies, and as we look to the future, it is evident that this trend will only accelerate. Emerging technologies promise to reshape the landscape of investing, enhancing both decision-making processes and operational efficiencies. One of the primary advancements expected is the increased use of machine learning algorithms that will refine predictive analytics for market trends. These algorithms will become more adept at identifying patterns in vast datasets, allowing investors to anticipate market movements with unprecedented accuracy.
Moreover, advancements in natural language processing (NLP) are set to revolutionize how financial analysts interpret news and social media sentiment. By systematically analyzing qualitative data, AI tools can provide insights into market psychology, enabling investors to make informed decisions based on public sentiment around particular stocks or sectors. This development will amplify the predictive power of AI-driven strategies, allowing for more nuanced approaches to portfolio management.
Furthermore, personalization in investment strategies is anticipated to become more prevalent, as AI can tailor recommendations based on individual investor profile and risk appetite. Robo-advisors are expected to evolve, leveraging AI to create customized investment portfolios that meet the specific financial goals and needs of each client. AI in Finance; This personalized approach not only enhances user experience but also democratizes access to sophisticated investment strategies traditionally available only to high-net-worth individuals.
Nevertheless, as with any technological advancement, it will be crucial for the finance industry to address potential challenges associated with AI adoption. AI in Finance; Ethical considerations, regulatory compliance, and data security will require ongoing vigilance. AI in Finance; The future of AI in investment strategies is poised to be one of continuous innovation, with the potential to redefine how investors engage with financial markets and manage their investments.